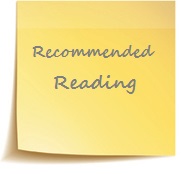
Previous Month | RSS/XML | Current | Next Month
WEBLOG
June 30th, 2022 (Revised: 7/2/2022) (Permalink)
When More is Less & Who are the Experts?
- Gary Smith, "Believe in Science? Bad Big-Data Studies May Shake Your Faith", Bloomberg, 4/26/2022
The cornerstone of the scientific revolution is the insistence that claims be tested with data, ideally in a randomized controlled trial. … Today, the problem is not the scarcity of data, but the opposite. We have too much data, and it is undermining the credibility of science.
Luck is inherent in random trials. In a medical study, some patients may be healthier. In an agricultural study, some soil may be more fertile. In an educational study, some students may be more motivated. Researchers consequently calculate the probability (the p-value) that the outcomes might happen by chance. A low p-value indicates that the results cannot easily be attributed to the luck of the draw.
How low? In the 1920s, the great British statistician Ronald Fisher said that he considered p-values below 5% to be persuasive and, so, 5% became the hurdle for the “statistically significant” certification needed for publication, funding and fame.
It is not a difficult hurdle. Suppose that a hapless researcher calculates the correlations among hundreds of variables, blissfully unaware that the data are all, in fact, random numbers. On average, one out of 20 correlations will be statistically significant, even though every correlation is nothing more than coincidence.
There's nothing magical about Fisher's 5% p-value for statistical significance. If, for some reason, you are checking so many variables for correlations, you need to use a lower p-value as the threshold for significance, say, 1%―this is known as adjusting for multiple comparisons. See the Multiple Comparisons Fallacy for more.
Real researchers don’t correlate random numbers but, all too often, they correlate what are essentially randomly chosen variables. This haphazard search for statistical significance even has a name: data mining. As with random numbers, the correlation between randomly chosen, unrelated variables has a 5% chance of being fortuitously statistically significant. Data mining can be augmented by manipulating, pruning and otherwise torturing the data to get low p-values.
The phrase "data mining" isn't always a pejorative: often it's used to refer to searching large sets of data for patterns. However, manipulating, pruning, and torturing data is usually called "p-hacking", which is always pejorative.
To find statistical significance, one need merely look sufficiently hard. Thus, the 5% hurdle has had the perverse effect of encouraging researchers to do more tests and report more meaningless results. Thus, silly relationships are published in good journals simply because the results are statistically significant. …
A team led by John Ioannidis looked at attempts to replicate 34 highly respected medical studies and found that only 20 were confirmed. The Reproducibility Project attempted to replicate 97 studies published in leading psychology journals and confirmed only 35. The Experimental Economics Replication Project attempted to replicate 18 experimental studies reported in leading economics journals and confirmed only 11.
A scientific result has no claim to our belief until it has been independently replicated, so beware of those who cite "the latest study" or "the most recent research", since the latest research has probably not been replicated.
I wrote a satirical paper that was intended to demonstrate the folly of data mining. I looked at Donald Trump’s voluminous tweets and found statistically significant correlations between: Trump tweeting the word “president” and the S&P 500 index two days later; Trump tweeting the word “ever” and the temperature in Moscow four days later; Trump tweeting the word “more” and the price of tea in China four days later; and Trump tweeting the word “democrat” and some random numbers I had generated.
I concluded — tongue as firmly in cheek as I could hold it — that I had found “compelling evidence of the value of using data-mining algorithms to discover statistically persuasive, heretofore unknown correlations that can be used to make trustworthy predictions.”
I naively assumed that readers would get the point of this nerd joke: Large data sets can easily be mined and tortured to identify patterns that are utterly useless. I submitted the paper to an academic journal and the reviewer’s comments demonstrate beautifully how deeply embedded is the notion that statistical significance supersedes common sense: “The paper is generally well written and structured. This is an interesting study and the authors have collected unique datasets using cutting-edge methodology.”
It is tempting to believe that more data means more knowledge. However, the explosion in the number of things that are measured and recorded has magnified beyond belief the number of coincidental patterns and bogus statistical relationships waiting to deceive us.
If the number of true relationships yet to be discovered is limited, while the number of coincidental patterns is growing exponentially with the accumulation of more and more data, then the probability that a randomly discovered pattern is real is inevitably approaching zero.
The problem today is not that we have too few data, but that we have too much data, which seduces researchers into ransacking it for patterns that are easy to find, likely to be coincidental, and unlikely to be useful.
Okay, but let's not throw out the "big data" with the p-hacking.
The following article is rather long, but worth reading as a whole, especially if you're interested in the problem of how to evaluate expertise. I have a great deal to say about that subject, and will frequently interrupt the author below to comment. So, you might want to read the whole article before perusing my commentary.
- Oliver Traldi, "With All Due Respect to the Experts", American Compass, 5/20/2022
A few weeks before Donald Trump’s inauguration as President, the New Yorker published a cartoon depicting a mustached, mostly bald man, hand raised high, mouth open in a sort of improbable rhombus, tongue flapping wildly within, saying: “These smug pilots have lost touch with regular passengers like us. Who thinks I should fly the plane?” The tableau surely elicited many a self-satisfied chuckle from readers disgusted by the populist energy and establishment distrust that they perceived in Trump’s supporters.
The cartoon is reminiscent of Plato's parable of the ship of state from The Republic:
Imagine…a ship in which there is a captain who is taller and stronger than any of the crew, but he is a little deaf and has a similar infirmity in sight, and his knowledge of navigation is not much better. The sailors are quarrelling with one another about the steering―every one is of opinion that he has a right to steer, though he has never learned the art of navigation and cannot tell who taught him or when he learned, and will further assert that it cannot be taught, and they are ready to cut in pieces any one who says the contrary. They throng about the captain, begging and praying him to commit the helm to them; and if at any time they do not prevail, but others are preferred to them, they kill the others or throw them overboard, and having first chained up the noble captain's senses with drink or some narcotic drug, they mutiny and take possession of the ship and make free with the stores; thus, eating and drinking, they proceed on their voyage in such a manner as might be expected of them. Him who is their partisan and cleverly aids them in their plot for getting the ship out of the captain's hands into their own whether by force or persuasion, they compliment with the name of sailor, pilot, able seaman, and abuse the other sort of man, whom they call a good-for-nothing; but that the true pilot must pay attention to the year and seasons and sky and stars and winds, and whatever else belongs to his art, if he intends to be really qualified for the command of a ship, and that he must and will be the steerer, whether other people like or not―the possibility of this union of authority with the steerer's art has never seriously entered into their thoughts or been made part of their calling.1
Traldi's criticism of the cartoon―and, presumably, of Plato's parable, as well, if he were to criticize it―is that it involves a weak analogy:
But what exactly is the joke here? Citizens in a democracy are not akin to airline passengers, buckled quietly into their seats and powerless to affect change, their destinations and very lives placed in the hands of professionals guarded by a reinforced door up front. Even brief reflection reveals the cartoonist’s analogy to be comparing like to unlike.
That none of us thinks we know better than a plane’s captain, yet we often think we know better than experts in matters of politics, suggests differences between those domains. And it highlights a vexing problem for modern political discourse and deliberation: We need and value expertise, yet we have no foolproof means for qualifying it. To the contrary, our public square tends to amplify precisely those least worthy of our trust. How should we decide who counts an expert, what topics their expertise properly addresses, and which claims deserve deference?
We all rely upon experts. When something hurts, we consult a doctor, unless it’s a toothache, in which case we go to a dentist. We trust plumbers, electricians, and roofers to build and repair our homes, and we prefer that our lawyers and accountants be properly accredited. Some people attain expertise through training, others through experience or talent. … In all these cases, our reliance on expertise means suspending our own judgment and placing our trust in another—that is, giving deference. But we defer in different ways and for different reasons. The pilot we choose not to vote out of the cockpit has skill, what philosophers sometimes call “knowledge how.” We need the pilot to do something for us, but if all goes well we need not alter our own beliefs or behaviors on his say so. At the other extreme, a history teacher might do nothing but express claims, the philosopher’s “knowledge that,” which students are meant to adopt as their own beliefs. Within the medical profession, performing surgery is knowledge-how while diagnosing a headache and recommending two aspirin as the treatment is closer to knowledge-that.
"Knowledge how"―or, more familiarly, "know-how"―is knowledge of how to do something, that is, a skill. "Knowledge that" is propositional knowledge: knowing the truth or falsity of a statement. Both types of knowledge are important, but philosophers have tended to ignore know-how in favor of propositional knowledge. Plato may have been the first to do so, since his teacher Socrates often used craftsmen as paradigms of knowledge.
Traldi now asks an important question concerning expertise:
…[H]ow are those without expertise to determine who has it? Generally, we leave that determination to each individual. A free society and the free market allow for widely differing judgments about who to trust about what, with credentialing mechanisms in place to facilitate signaling and legal consequences for outright fraud. …
In the public square, we have historically placed our trust in a similar phenomenon, the wisdom of crowds. In the early 19th century, the Marquis de Condorcet codified the thesis that citizens who know less than experts can together generate a system that knows more. Condorcet’s Jury Theorem2 demonstrates a simple consequence of the law of large numbers: If voters are more likely to vote correctly than incorrectly and their votes are statistically independent of each other, then as the number of voters increases, the probability that voters get the right result approaches 100%. In large enough numbers, thinking for themselves, the vox populi will make the right decisions.
There are a lot of problems with applying this theorem to juries, let alone to democracies as a whole: What is it to "vote correctly" on a jury? What basis do we have for thinking that individual jurors are more likely to vote correctly than incorrectly? Isn't it likely that some jurors are more likely to vote correctly, but others incorrectly? Moreover, the same theorem leads to the conclusion that if the jurors are more likely to vote incorrectly, then as the number of jurors increases, the probability of an incorrect verdict approaches 100%.
If we took the application of this theorem to juries seriously, then we should increase the size of juries, since the larger the jury the more likely that its judgment will be correct―always assuming that each juror is more likely to be correct than incorrect. While contemporary juries are usually twelve, but sometimes half that, the juries in ancient Athens were much larger. In fact, 500 jurors convicted Socrates and sentenced him to death3.
Also, the assumption that the votes of jurors or citizens of a democracy are statistically independent is certainly wrong, at least as trials and elections are currently conducted. For the votes of jurors to be independent, it would have to be the case that no juror influenced the vote of any other, but we expect that juries will deliberate before voting. If we took the theorem seriously, we would need to keep the jurors separate so that they could not influence one another. Similarly, we expect that citizens of a democracy will discuss and debate before an election, whereas the theorem would indicate that this should be forbidden.
So, Condorcet's theorem, while obviously true as a result in probability theory, simply doesn't apply to juries or electorates made up of fallible human beings. If we want to get along without experts in trials and elections, we'll have to look elsewhere.
This rational populism has not been sitting well with the expert class, which finds democracy an inconvenient obstacle to technocratic rule. Thus the recent emphasis on “cognitive biases,” which treat the typical citizen as not only a non-expert himself, but also incapable of identifying the real experts or aggregating his opinions with other non-experts to achieve a reasoned result. There is confirmation bias, negativity bias, status quo bias, “tribalism,” and so on. Particularly en vogue are the “implicit biases” of identity and diagnoses of “racial resentment” and the “authoritarian personality”, which were used to explain the results of the 2016 election. Constructs like these provide the backdrop for expert dismissals of disfavored political views. Alongside the technically framed analyses of how people misprocess correct information comes an assumption that they are also cheaply programmable, easily gulled by puppeteer propagandists and “Fake News.” Only the experts, then, can tell us who is truly an expert. Only someone untrustworthy would not trust them.
Many straw men are assaulted in this passage. At the very least, it's a caricature of those of us who take cognitive biases seriously. I, for one, accept the existence of biases, but reject the notion that anyone should just blindly trust experts. For one thing, cognitive biases are human biases, which means that experts suffer from them as much as non-experts. Secondly, no one is expert in everything, so we're all non-experts in most fields. Finally, there are no experts in identifying experts, but suppose that there were. How is the non-expert supposed to identify such an expert? The problem of identifying expertise is a problem for all of us, expert and non-expert alike, and "trust the experts" is no solution. Who are the experts we're supposed to trust? That's the problem!
…[T]he expert critique of common sense and the crowd’s wisdom is wrong. Ironically, the expert critique more effectively serves as a critique of experts. More so than the population, they appear susceptible to motivated reasoning and belief cascades. …[C]ommon sense remains an essential part of the expert’s arsenal. The apparent expert who abandons it may end up worse off than the non-expert.
While experts are human, and thus as susceptible to human biases as anyone, I see no evidence here that they are more so.
Experts are also susceptible to processes that arbitrarily reinforce an unexamined consensus, both ex ante and ex post. … The consensus view is a prerequisite for qualifying as an expert, not a considered consequence of one’s genuine expertise. …
There's a third possibility: that one must accept the consensus view to qualify as an expert, and that it is also an expert conclusion. For instance, I doubt seriously that a person who believes that the earth is flat would be able to get a doctorate in astronomy from an accredited institution.
Once qualified, experts remain susceptible to the related phenomenon of belief cascades as new questions emerge, because they place their standing at risk if they depart from the views of fellow experts. (After all, what could threaten the enterprise more than the revelation that expertise does not dictate a particular conclusion?) When one expert, or a small number of experts, expresses a certain view—especially on a politically charged topic—that view quickly propagates through social networks both formal and informal, public and private, and becomes widely held on the assumption that experts can be trusted. What appears a broad-based consensus among people who have thought about the issue is really only the overamplified view of the few people who have thought about it at all.
This appears to be a description of the phenomenon of group think, but the non-expert population is just as likely to be affected by it. Scarcely a day passes without some faddish delusion spreading like wildfire via "social" media.
Moreover, experts do depart from the views of their fellows, as we have seen the last couple of years. They do run some risk of having their careers damaged, and some experts are probably silent for fear of such risks. This has happened throughout scientific history, and it's a shame that it still happens. Nonetheless, we have seen experts brave those risks, and some have been vindicated in short order.
Yet another problem for our experts is that the source, nature, and relevance of their expertise is often ill-defined. … Even when expertise is genuine, disciplines and professions, along with their practitioners, seem determined to overextend its breadth for purposes of laundering their personal, non-expert opinions under their expert brand. …
I've left out here some examples of experts opining outside of their field, not because I disagree with the examples―I don't―but you can read them for yourself. Also, there's no doubt that some experts tend to over-extend themselves, but this is one of the easiest problems for the non-expert to spot: all that you need to know is what the expert's domain of expertise is, and to what domain the expert's opinion belongs. If the two domains are not the same, then the expert's opinion is no better than any other non-expert.
…[T]his…illustrates perhaps the modern expert’s biggest problem: To become visible enough that non-experts can find him, he must proffer his views through Twitter, on talk show interviews, and in essays for magazines…. Such platforms select for certain experts, and certain views. Laypeople encountering an expert “in the wild” have no reason to think that he is representative of his discipline and every reason to think the opposite. …
The public will never be able to assess the validity of expertise on a case-by-case basis. Trying yields widely varied conclusions and thus eliminates any common starting point from which to conduct public debates—roughly the situation today. Assessing apparent expertise requires knowledge of a field’s inner workings, something almost no one has the time or inclination to learn. From the outside, it is difficult to infer what dogmas might contaminate a discipline’s standard training or what pressures might distort processes of hiring, promotion, and socialization.
This sounds discouraging, but see the next sentence:
However, some general heuristics and defaults might provide a basis for at least some agreement. First, a simple conflict-of-interest standard would make sense. Look at what people gain from giving their views, and from whom they gain it. Someone who stands to gain more personally from one view than from another should not be entitled to deference when offering the former. That does not mean the view is wrong, only that it must be defended on its merits rather than based on the identity of the speaker. …
Now we're getting somewhere. Enough with the expert-bashing; it may be fun, but it's not advancing us towards a solution of the problem.
I agree that we should watch out for conflicts of interest, but would add that every view must be defended on its merits, and not on the identity of the person who advances it. Even experts―especially experts―should defend the views they advance in this way, and not simply ask that we trust them.
Second, political stances should be inherently suspect. Experts can offer knowledge useful in evaluating the values-laden tradeoffs of politics and public policy, but that expertise does not make their judgment superior to that of any other citizen, and certainly not the democratic determination of a large group of citizens. …
Third, we should be far more skeptical of claims of knowledge-that expertise than of knowledge-how. In the latter case, people’s claims of expertise can be substantiated by their ability to deliver objective results. The surgeon with a track record of successful surgeries is easily distinguishable from the charlatan with none. Knowledge-that experts, by contrast, are laying claim to the truth. Sometimes they have it, and are guiding us as reliably as a pilot. Other times they are simply taking us for a ride.
It's true that one way to check the know-how of supposed experts is by examining their work, but this is not always easy since it may require knowledge to tell the difference between good and bad work. Also, it is often possible to check the propositional knowledge claims of alleged experts, especially when they make predictions. If supposed experts make false predictions, we should mistrust their future predictions. In addition, false predictions call into question the theories that they're based on.
Despite Traldi's pessimism, this article shows that it is possible to make progress in identifying both expertise and when to rely on it. This will always be a difficult task, and mistakes are inevitable, but we are all forced by the complexities of the world to rely on the knowledge of others. Even Traldi admits this when he's not bashing experts. So, there's no alternative to doing the best that we can and, hopefully, we will muddle through.
The first article is by Gary Smith, the author of Standard Deviations, a book I've reviewed and recommend―see the Book Shelf page in the Main Menu to your left.
Notes:
- Plato. The Republic, 488A-489A; Jowett's translation.
- Eric W. Weisstein, "Condorcet's Jury Theorem", Wolfram's MathWorld, accessed: 6/29/2022.
- Douglas O. Linder, "Criminal Procedure in Ancient Greece and the Trial of Socrates", Famous Trials, accessed: 6/29/2022.
Disclaimer: I don't necessarily agree with everything in these articles, but I think they're worth reading as a whole. In abridging them, I may have changed the paragraphing and rearranged the order of the excerpts in order to emphasize points.
June 26th, 2022 (Permalink)
Inflation and "Record-High" Gas Prices
Inflation in America increased to 8.6% last month, a level that we haven't experienced for forty years. An important aspect of that inflation is the price of gasoline, which has risen at a rate much higher than the overall rate of inflation, increasing by nearly 50% over the last year1. You don't need the news media to tell you that the cost of gasoline has gone up a lot this year: just fill up your gas tank and you'll be painfully aware of it. Nonetheless, the news media keep referring to "record" or "record-high" gasoline prices2. In what sense is the price a "record"?
The claim that gas prices are setting new records is based on an average of prices nationwide compiled by the American Automobile Association (AAA). According to the AAA, the current national average price for a gallon of regular unleaded gasoline is $4.903. The "record high" was actually set on the 14th of this month at $5.016, so it's not now at a record-setting price, but only about a dime away.
What the news media don't usually mention is that AAA's average measures nominal gas prices, that is, simply the price on the pump unadjusted for inflation. Inflation is money losing value over time―which it's been doing unusually fast for the last several months―so that a dollar today is not worth what it was yesterday. A dollar this year will buy less gas than it would have last year, let alone ten, fifty, or one-hundred years ago.
Given inflation, comparing prices from many years apart is comparing apples to oranges or, for a less hackneyed and fruity analogy, it's like comparing prices in American dollars with prices in Canadian dollars without taking the exchange rate into consideration. "The past is a foreign country", as L. P. Hartley wrote4. So, to compare today's prices to those many years ago, you should adjust for inflation5.
Adjusting for inflation, the previous record was set in 2008, when prices averaged $4.116, which is $5.49 in today's dollars7. Inflation is running so high currently that it's entirely possible that we'll see inflation-adjusted prices exceed $5.49 in the near future, which would be a real as opposed to a merely nominal record price.
Notes:
- Aimee Picchi, "Inflation surged 8.6% over the last year — fastest since 1981", CBS News, 6/10/2022.
- For just one example: Chris Isidore, "Average US gas price hits $5 for first time", CNN, 6/13/2022.
- Gas Prices, American Automobile Association, accessed: 6/26/2022.
- L. P. Hartley, The Go-Between (1953), prologue. From Bartlett's Familiar Quotations, Justin Kaplan, General Editor (1992, 16th edition), p. 692.
- If you want to adjust prices for inflation, see: Elizabeth B. Appelbaum, "The Consumer Price Index and Inflation - Adjust Numbers for Inflation", Mathematical Association of America, 12/2004. The math is not difficult, but you can use the Bureau of Labor Statistics' online calculator, instead: "CPI Inflation Calculator", Bureau of Labor Statistics (BLS).
- Sarah Hansen, "5 Facts That Show How Painful Gas Prices Are Now", Money, 6/7/2022.
- I used the BLS calculator to adjust for inflation, with June of 2008 for the previous record, since the source gave the nominal price only for the summer of that year and not the month. I also used May of this year, which is the last month for which data is available, for the current price.
June 9th, 2022 (Permalink)
How to Get a Correction or Retraction in Ten Easy Steps
If, in the course of amateur fact-checking, you discover a factual error in a publication, what should you do then? You've received the benefit of not being misled by the error, but what about other readers or viewers who will not make the effort to check the mistaken claim? My suggestion is that you request a correction or retraction from the source that made the mistake, so that unwary people will not be misinformed.
What's the difference between a correction and a retraction? In a correction, the article itself may be edited to remove or correct the error, and usually a notice at the top or bottom of the article will notify the reader that a correction has been made. Sometimes, the article itself will not be corrected, but a correction appended at the beginning or end. Other times, the notice of a correction will appear on a separate corrections page, though this is not good internet practice.
A retraction is more drastic than a correction, and you are unlikely to get one for a single mistake unless it undermines the thesis of the article. In a retraction, the entire article will be removed, with perhaps a note replacing it that explains the retraction. Articles are most likely to be retracted for extensive plagiarism or fabricated information rather than easily correctable errors.
- Don't request a correction over a difference of opinion: Only make such a request when a publication has committed a checkable factual error. If you're not sure whether something is a matter of opinion or of fact, then review the previous entries in this series on fact-checking1. If you're not sure whether something is a factual error, or whether it's a factual error, then don't ask for a correction. Be sure that you're on solid ground before contacting a publication. If it is a difference of opinion, then there are many ways that you can challenge the publication's claims: send a letter to the editor, add a comment to the article, or write and publish your own response. Don't waste your and the publication's time by demanding the correction of an opinion.
- Request a correction first: Have the courtesy to contact a publication and request a correction or retraction before you publicly criticize it for a mistake. Give it a chance to do the right thing. This warning includes adding a public comment to an article if the publication allows such a thing, so don't use such comments as a way to try to get a mistake corrected or an article retracted.
- Be polite: If you want to get a correction or retraction, don't insult the readers of your request or the publication for which they work. Don't call them ignoramuses, fools, or worse―they may actually be ignorant fools, but don't say so. Assume that they want to get it right. Don't use sarcasm to suggest that they are idiots, or that it is unlikely that they will honor your request―there's no better way to get them to ignore you. If you violate this rule your request is most likely to end up in the trash.
- Don't curse: This, of course, is part of politeness, but it may need special emphasis nowadays. If you curse at the person reading your request or the publication the person works for, your request will justifiably go in the trash.
- Be specific: Describe the error you want corrected exactly and precisely. If you just have some vague feeling that an article is mistaken, then you're not going to get a correction anyway, so don't bother asking for one.
- Be able to prove your case: Don't request a correction or a retraction unless and until you can prove the publication committed a mistake beyond a reasonable doubt. This is an unfair standard, but it is likely to be the one that you'll be held to. If there's any way for a publication to wriggle out of the need to correct or retract something, it will usually try to do so. Publications do not like to issue corrections or, especially, retractions. So, you need to have such a solid case that there's no wiggle room. If you can't prove your case, you can still request a correction or retraction, but don't expect one.
- Don't hold your breath: As I mentioned above, publications don't like to make public corrections, let alone retractions. This is true―perhaps especially true―of even the most prestigious and reputable institutions. So, don't be surprised if your request is silently rejected.
- If your request is granted, thank the publication and its representative: This, of course, is also a matter of politeness. However, we need to encourage publications to admit error and correct the public record, so thank them when they do so! Anybody can make a mistake, but they did the right thing despite the likelihood of public embarrassment, so they deserve praise and reward for doing so.
- If your request is denied or ignored, don't demand that your subscription be cancelled: In the lapidary words of William F. Buckley, Jr.: "Cancel your own goddam subscription!"2
- Go public: If you did all of the above, and the publication still does not correct or retract its mistake, publicly embarrass it! There are, of course, many ways that you can lay your case before the public. About the only thing a publication likes less than issuing corrections or retractions is being publicly shamed for getting something wrong. If the publication allows comments to its articles, you can add your correction of it to the comments. You can contact a rival publication, especially one with a different political slant, which may be eager to point out the mistakes of its competitor. Just as we need to reward those who do the right thing, we need to punish those who do not. Let's make it easier and less painful to admit error than not to.
Notes:
- In chronological order:
- Why You Need to be Able to Check Facts, 9/8/2020
- Fact-checking Vs. Nit-picking, 10/20/2020
- Four Types of Misleading Quote, 11/27/2020
- News Sources Vs. Familiar Quotations, 12/4/2020
- Rules of Thumb, 1/2/2021
- A Case Study, 2/4/2021
- Reliable Sources, 3/2/2021
- Fact-Checking: What is a fact?, 4/29/2021
- Sources for Fact-Checking: Primary, Secondary & Tertiary, 5/6/2021
- Fact-Checkers ≠ Lie-Detectors, 8/27/2021
- Fact Checks, Vast Majorities, and Outright Falsehoods, 10/16/2021
- "Everyone is entitled to his own opinion, but not his own facts.", 11/23/2021
- Fact Checking the Future and the Future of Fact Checking, 12/5/2021
- William F. Buckley, Jr., Cancel Your Own Goddam Subscription: Notes and Asides from National Review (2007)
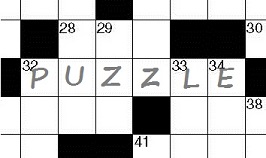
June 7th, 2022 (Permalink)
Crack the Combination II
The combination of a lock is three digits long. Here are some incorrect combinations:
- 054: Two of the digits are correct, one is in the right position, but the other is in the wrong place.
- 754: Two digits are correct but both are in the wrong positions.
- 742: Two of the digits are correct, one is in the right position, but the other is in the wrong place.
Can you determine the correct combination from the above clues?
047
Explanation: 4 must be part of the combination since this is the only digit that clues 1 & 3 share. 4 is not in the final position, since it is in that position in clue 2. 5 is not in the middle position, by clues 1 & 2, since it cannot be in both the right and wrong position, so 0 must be in the first position. Thus, 5 is not in the combination, by clue 1. Therefore, the full combination must be 047, by clue 2 and previous results.
WARNING: May cause brain-teasing.
June 4th, 2022 (Permalink)
Cite or Site?
A report in the The New York Times from fifty years ago about a water main break contained the following sentence: "A portable toilet unit on the construction cite also fell into the hole in the street.1" The toilet, of course, was on a construction site.
"Cite" is a verb, most commonly occurring in scholarly writing, which means to point to a source of supposed evidence for a claim or a quote2. In this weblog, I often cite sources for the information and quotes that I write about.
In contrast, "site" is usually a noun meaning "place", as in "web site" or "construction site"3. Given that the two words are pronounced identically, they are easy to confuse. Oddly, only two of the books I usually check, and sometimes cite, warn against such confusion4, though it seems to be a common error. In my experience, the most common mistake is to misspell "cite" as "site", though the confusion obviously can go in the opposite direction, witness the Times example.
Since "cite" is a verb and "site" is usually a noun, it's possible that a grammar checking program will catch confusion of one for the other. However, "site" can also be used as a verb meaning "to place", which means that a grammar checker may not catch it. My old copy of Microsoft's Word program flagged "cite" in the above example, and one online program automatically changed it to "site", though another did not. So, I would suggest that you test whatever program you usually use to see whether it would catch this mistake. If not, you can either upgrade your program or add this distinction to your mental software.
Notes:
- Martin Gansberg, "Subway Flooded by a Broken Main", The New York Times, 9/28/1970. I found this example in the following article: "'Cite' vs. 'Site' vs. 'Sight'", Merriam-Webster, accessed: 6/4/2022.
- "Cite", Cambridge Dictionary, accessed: 6/4/2022. For instance, this note cites the entry for "cite" in the online Cambridge Dictionary.
- "Site", Cambridge Dictionary, accessed: 6/4/2022.
- They are:
- Mignon Fogarty, Grammar Girl's 101 Misused Words You'll Never Confuse Again (2011), p. 29
- Robert J. Gula, Precision: A Reference Handbook for Writers (1980), p. 209
June 2nd, 2022 (Revised: 6/4/2022) (Permalink)
The Signal in the Noise
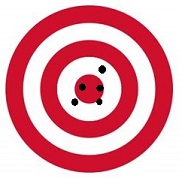
Quote: "To understand error in judgment, we must understand both bias and noise. Sometimes…noise is the more important problem. But in public conversations about human error and in organizations all over the world, noise is rarely recognized. Bias is the star of the show. Noise is a bit player, usually offstage. The topic of bias has been discussed in thousands of scientific articles and dozens of popular books, few of which even mention the issue of noise. This book is our attempt to redress the balance."1
Title: Noise
Comment: I was put off this book for several months by the title. While it's obvious that it's not a book about "noise" in the everyday sense of the word, it's not clear what it is about. There is a technical meaning of "noise" from information theory2, which is usually contrasted with "signal". Initially, based on the title, that's what I thought the book must be about, but at least two of the authors did not seem to be connected to information theory.
The phenomenon discussed in this book seems not to be "noise" in the information theory sense. "Noise", in that sense, refers to interference in a message, that is, something from outside that corrupts the message. For instance, static on a telephone line is "noise", and so is literal noise when you are trying to hold a conversation in a loud, crowded room. The message is the "signal", and "noise" is anything that interferes with or corrupts the signal.
In the Introduction, the authors use diagrams to explain what they mean by "noise". Suppose that we're shooting a gun at a target; the first target, shown to the above right, is what we were aiming at, that is, to have all of the bullet holes grouped as closely together as we can get them in or near the bull's-eye.
In contrast, the second target to the above right shows that we have consistently missed the bull's-eye, though at least the holes are close together.
In the third target, above right, the holes are at least centered on the target, but they are widely spaced with only one close to the bull's-eye.
Finally, the fourth and last target, right, is the worst performance. The holes are not centered on the bull's-eye and are widely dispersed.
There are two distinct phenomena demonstrated in these four diagrams, which I'll call "accuracy" and "precision". Accuracy is the closeness of the bullet holes to the bull's-eye, whereas precision is the closeness of the bullet holes to each other. So, in the first target, we see both accuracy and precision: the bullet holes are close to the bull's-eye, and they are grouped close together. In the second target, we have precision, because the holes are closely grouped, but the shooting is inaccurate. The third target shows accuracy in that the holes are centered on the bull's-eye, but the shooting is imprecise. Finally, the fourth target shows neither accuracy nor precision: the holes are spread out and uncentered on the bull's-eye.
Interestingly, I recently purchased a book published ten years ago that uses the same target analogy:
…[P]recision is not accuracy. Let's go to a rifle range. Accuracy means that the average location of all of our shots is in the bull's-eye; precision means all of our shots hit close together. If all our shots hit the bull's-eye, then our shooting is both precise and accurate. If all of our shots cluster in a dime-size hole far from the bull's-eye, then our shooting is precise but not accurate. If all of our shots are centered on the bull's-eye but evenly distributed over the entire target, then our shooting is accurate (on average) but not precise.3
The only thing missing from this description is the last target, in which the shooting is both inaccurate and imprecise.
In contrast to "accuracy" and "precision", the New Book's terms are negative, that is, they call inaccuracy "bias" and imprecision "noisiness". So, in those terms, the first and third diagrams show unbiased shooting, and the third and fourth are noisy. The first diagram has unbiased shooting with little noise, the second is biased but not noisy; the third is unbiased but noisy; and the last is both biased and noisy.
As indicated by the title and the Quote from the Introduction, above, the book is concerned with noise rather than bias, that is, with imprecision instead of inaccuracy.
Subtitle: A Flaw in Human Judgment
Comment: How is noise a "flaw" in human judgment? Noise has multiple causes, many of which come from outside of us. I could see calling it a "problem" for human judgment, but calling it a "flaw" makes it sound as though it is a mistake that comes from within. I guess I'll see whether the authors make good on the subtitle, or perhaps it was foisted on them by the publisher.
Authors: Daniel Kahneman, Olivier Sibony & Cass R. Sunstein
Comment: Kahneman is, of course, one half of the team of Kahneman & Tversky, famous for its work on cognitive biases. I'm unfamiliar with the second author. Cass Sunstein is the co-author, among other works, of Nudge, which I was highly critical of shortly after it came out almost fifteen years ago4. That book was about trying to exploit human biases for allegedly paternalistic reasons, and I hope this book is not about trying to manipulate people in order to reduce noise―see the General Comment, below.
Summary: According to the Introduction5, the book is divided into six parts on the following topics:
- The difference between bias and noise: it argues that some areas of human judgment are noisy―I, for one, don't need any convincing. In fact, I would go farther by pointing out that some areas are so noisy that the results are random. See Philip Tetlock's work on political prognostication6, for example.
- Occasion noise: when an individual person's judgments are unreliable.
- Predictions, which are notoriously noisy: for example, consider the predictions over the last couple of years of the course of the coronavirus epidemic; they were both biased and all over the place, that is, noisy. If plotted on a target, the results would look like the fourth target, above.
- Human psychology: the sources of noise in individual judgments.
- Practical advice on noise abatement.
- What is the right noise level? The authors suggest that it is not zero noise, which is probably right at least for practical reasons.
The Blurbs: The book is favorably blurbed by Robert Cialdini, author of Influence; Annie Duke, author of Thinking in Bets; Jonathan Haidt; freakonomist Steven Levitt; and the aforementioned Philip Tetlock.
Date: 2021
Comment: This book is from last year, so it's not brand new, but I'm just getting around to reading it for reasons explained in the first Comment, above.
General Comment: Keeping in mind that I've read only the Introduction so far, my only misgiving about this book is that it appears to emphasize precision over accuracy, and I don't think that noise reduction should be an end in itself. Certainly, if we find ourselves in the situation represented in the third target, we would like to reduce the noise so that we get closer to the first target. However, suppose that the situation we find ourselves in resembles the fourth target, where there is both bias and noise, which is the most common situation. Why should we want to go from the last target to the second one, that is, reducing noise without improving accuracy?
The situation illustrated by the second target may actually be a worse one than the last target in that the precision of the shooting may give a false impression of accuracy. As the authors point out7, if we look at the backs of the targets we can't tell the difference between the first and second, which is often the situation we're in since we don't know exactly where the bull's-eye is.
In particular, I'm skeptical about the value of a program to reduce the noise in social and political judgments, since this may result in a misleading conformity. The easiest way to reduce imprecision in judgment is to compel people to conform through social pressure such as shunning and threats to one's livelihood or even life. Such pressure can create a factitious "consensus", such as that about the lab leak hypothesis or Hunter Biden's laptop.
These are just preliminary questions and doubts that occurred to me as I read the Introduction and, hopefully, the book will address and answer them adequately.
Disclaimer: I haven't finished reading this book yet, so can't review or recommend it. However, its topic interests me, and may also interest Fallacy Files readers.
Notes:
- Pp. 5-6. All page references are to the new book.
- George Markowsky, "Information Theory", Encyclopaedia Britannica, accessed: 5/10/2022.
- Lawrence Weinstein, Guesstimation 2.0 (2012), p. 344. I adopted the term "precision" from this passage in place of "reliability".
- See: Fallacy Files Book Club: Nudge, Introduction, 5/12/2008, and subsequent installments.
- Pp. 8-9.
- Philip Tetlock, Expert Political Judgment (2005).
- P. 4.