WEBLOG
Previous Month | RSS/XML | Current | Next Month
September 30th, 2013 (Permalink)
A Headline and a Reminder
Sun Sued in Puerto Rico by Conservation Trust
It's the global warming, stupid!
Source: Robert Goralski, compiler, Press Follies (1983)
- The Fallacy Files is a self-supporting website. If you value this site, please consider donating via the "Donate" button to your right. Thank you for your support!
September 28th, 2013 (Permalink)
A New Puzzle Book and a New Puzzle
Logician Raymond Smullyan is out with a new puzzle book called, appropriately enough, The Gödelian Puzzle Book: Puzzles, Paradoxes & Proofs. To celebrate, here's a new puzzle―actually, it's an old puzzle, but I haven't used it here before so it's new to us.
Three sisters are having a disagreement:
Lorie: What Alice is going to say is false!
Alice: What Edith is going to say is false!
Edith: What Lorie and Alice both just said is false!
Which, if any, of the three sisters is telling the truth?
September 26th, 2013 (Permalink)
12 Types of Study You Need to Know About!
Brian Dunning of "Skeptoid" has a useful rundown of different types of statistical study in the form of a podcast with accompanying transcript (see the Source, below). If you've ever wondered what a "cohort" study is, or what's the difference between a "cross-sectional" study and a "longitudinal" one, now you can find out. There are brief explanations of at least a dozen different types. Check it out.
Source: Brian Dunning, "An Enthusiast's Primer on Study Types", Skeptoid Podcast, Skeptoid Media, 9/24/2013
September 24th, 2013 (Permalink)
Doublespeak Dictionary
Apparently, lobbyists have an image problem, so what can they do? According to an opinion piece in The New York Times (see the Source, below), they're thinking about "rebranding" lobbying. They can't change what they do, but they can change what they call it. One suggestion is to replace "lobbyist" with "government relations professional". This has a lot to be said for it: it's unfamiliar and when people first hear it they won't know what it means; it's so general in meaning that no one will be able to figure out what they do; and it's so long that it would probably be abbreviated as "GRP", which is even less revealing than the full title. Eventually, though, people will figure out that a GRP is just a glorified lobbyist, and then they'll need to "rebrand" again.
Source: Francis X. Clines, "Lobbyists Look for a Euphemism", The New York Times, 9/21/2013
Previous entries in the doublespeak dictionary: 2/24/2005, 7/2/2006, 7/17/2006, 3/25/2008, 9/25/2008, 3/14/2009, 3/29/2009
September 20th, 2013 (Permalink)
Blurb Watch: GMO OMG
An ad for the new anti-genetically modified food documentary GMO OMG has it all―well, not quite all, but it does have examples of three of the common tricks of the blurbing trade:
- There's the one-word blurb: "Illuminating!"-Ernest Hardy, THE VILLAGE VOICE. Of course, there's no exclamation point in the original and the review includes some less flattering words in the full context, calling the movie "a documentary that is by turns exasperating, illuminating, and intentionally infuriating."
Source: Ernest Hardy, "GMO OMG: Who Controls the World's Food Supply?", The Village Voice, 9/11/2013
- Then, there's the four stars from a review―in Eater of all things, but then it's a documentary about food. Not surprisingly, the ad doesn't mention that the four stars are out of a possible five.
Source: Joshua David Stein, "Review: The Inconvenient Truth of GMO OMG", Eater, 9/3/2013
- Finally, there's the blurb that pulls a passing positive comment out of a mostly-negative review: "Provides a gentle, flyover alert to obliviously chowing-down citizens…without hectoring and with no small amount of charm."-Jeannette Catsoulis, THE NEW YORK TIMES. Here's the context:
"GMO OMG", Jeremy Seifert’s plain-folks primer on genetically modified food['s]…central concern―that Americans are inadequately informed about the corporate manipulation of the food supply―may be valid, but its reliance on cute-kiddie silliness does little to fill that void. Even children as photogenic as Mr. Seifert’s are no substitute for rigorous research. … But to the informed consumer hoping for greater elucidation, Mr. Seifert’s partisan, oversimplified survey falls short. Approaching this extremely complicated topic with the air of an innocent seeker, he barely touches on the science behind the genetically modified organisms (G.M.O.) that are introduced into the food, focusing instead on…a study that has since been scientifically questioned. … In fairness to Mr. Seifert, that’s a lot to cram into 90 minutes. And if his not unreasonable goal is to provide a gentle, flyover alert to obliviously chowing-down citizens, then he does so without hectoring and with no small amount of charm. I told you those kids were cute.
Source: Jeannette Catsoulis, "Microscope on the U.S. Food Supply", The New York Times, 9/12/2013
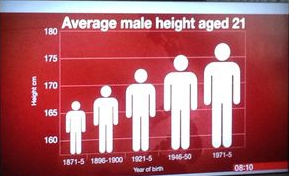
September 15th, 2013 (Permalink)
A Charts & Graphs Pop Quiz
If you've been following along in this series of entries on misleading charts, here's a chance to test what you've learned. If you haven't, you can catch up by reading the previous entries listed below.
When you're ready, take a look at the graph shown to the upper right. This chart commits at least one of the sins explained in the previous entries in this series. Your mission, should you decide to accept it, is to identify which sin or sins it commits. When you think you know the answer, click "Answer" below and find out if you're right.
Previous entries in this series:
- The Gee-Whiz Line Graph, 3/21/2013
- The Gee-Whiz Bar Graph, 4/4/2013
- Three-Dimensional Pie, 5/5/2013
- The 3D Bar Chart, Part 1, 6/3/2013
- The 3D Bar Chart, Part 2, 7/11/2013
- The One-Dimensional Pictograph, 8/1/2013
September 10th, 2013 (Permalink)
8 Tools that Help You Think Better!
Philosopher Daniel Dennett's latest book is Intuition Pumps and Other Tools for Thinking. Most of the "tools for thinking" that Dennett describes will be of interest primarily to other philosophers since they concern such philosophical matters as meaning, consciousness, and free will. However, the first group of tools are "a dozen general thinking tools", which should be of interest to anyone―at least, anyone who thinks. I've got to get in on this "listicle" craze, so here's a list of eight of the tools:
- Making Mistakes: It's a commonplace that we learn from our mistakes; more accurately, we can learn from our mistakes if we're willing to admit that we've made them. Unfortunately, people are reluctant to admit to error, because nobody likes to be wrong, and there's often a social price to be paid for admitting to a mistake. Making a mistake is nothing to be proud of, but being brave enough to admit it is. As Dennett writes:
The chief trick to making good mistakes is not to hide them―especially not from yourself. … The fundamental reaction to any mistake ought to be this: "Well, I won't do that again!" … So when you make a mistake, you should learn to take a deep breath, grit your teeth, and then examine your own recollections of the mistake as ruthlessly and as dispassionately as you can manage. It's not easy. The natural human reaction to making a mistake is embarrassment and anger (we are never angrier than when we are angry at ourselves), and you have to work hard to overcome these emotional reactions. (Pp. 22-23)
- Reductio ad Absurdum:
The crowbar of rational inquiry, the great lever that enforces consistency, is reductio ad absurdum―literally, reduction…to absurdity. You take the assertion or conjecture at issue and see if you can pry any contradictions (or just preposterous implications) out of it. If you can, that proposition has to be discarded or sent back to the shop for retooling. (P. 29)
- Sturgeon's Law: I was surprised to see "Sturgeon's Law"―which is that 90% of everything is crud―listed here, since it seems to be more an empirical rule of thumb than a thinking tool. However, Dennett's point is not to waste time attacking straw men; in controversy, attack your enemy's strong points, not their weak points―that is, the crud.
- Occam's Razor: This one's obvious.
- Occam's Broom: This one's actually not a thinking tool, but "the process in which inconvenient facts are whisked under the rug by intellectually dishonest champions of one theory":
This is…an anti-thinking tool, and you should keep your eyes peeled for it. The practice is particularly insidious when used by propagandists who direct their efforts at the lay public, because like Sherlock Holmes's famous clue about the dog that didn't bark in the night, the absence of a fact that has been swept off the scene by Occam's Broom is unnoticeable except by experts…the lay reader can't see what isn't there.
How on earth can you keep on the lookout for something invisible? Get some help from the experts. … Conspiracy theorists are masters of Occam's Broom, and an instructive exercise on the Internet is to look up a new conspiracy theory, to see if you (a nonexpert on the topic) can find the flaws, before looking elsewhere on the web for the expert rebuttals. …[E]ven serious scientists cannot resist "overlooking" some data that seriously undermine their pet theory. It's a temptation to be resisted, no matter what. (Pp. 40-41)
I'm not thrilled with the name "Occam's broom" because it's not fair to poor old Occam, but it does open up a world of possible new names for things: how about "Occam's toothbrush", "Occam's pencil sharpener", or "Occam's chainsaw"? I usually call the results of sweeping with Occam's broom "one-sidedness" or "slanting", and even have a fallacy entry under those names (see under the new alphabetical list of fallacies to your left). This is probably the most insidious of all fallacies because it is often undetectable unless you happen to already know about the facts that have been left out.
- The "Surely" Operator:
When you're reading or skimming argumentative essays…here is a quick trick that may save you much time and effort, especially in this age of simple searching by computer: look for "surely" in the document, and check each occurrence. Not always, not even most of the time, but often the word "surely" is as good as a blinking light locating a weak point in the argument…. (P. 53)
Of course, this is true of other words and phrases, such as "obviously", "no doubt"―and "of course"! These and similar words and phrases are called "assuring terms" (see the Source, below) because they assure us that something is true without actually giving any reason to think that it is. As Dennett notes, there's nothing necessarily wrong with using assuring terms, but they can be misused to conceal weak premisses. Every argument has to stop somewhere, and to be persuasive that somewhere needs to be a premiss that the audience will accept as true, and even obvious.
- Rhetorical Questions: A rhetorical question is, of course, a statement in the guise of a question. Dennett seems to think that rhetorical questions are disguised Reductio ad Absurdum arguments, and perhaps some are, but I don't think that's the main problem with them. Rather, the most common problem with such questions is that some people use them to sneak in assertions that they don't then defend―there's a good example of this kind of use in the entry for "loaded question" (see the alphabetical list of fallacies to your left). "I'm just asking questions", they assert. No, they're not! A rhetorical question is not just a question; it's also an assertion. Be on the lookout for such questions and don't let them get away with it.
- "Deepities":
A deepity is a proposition that seems both important and true―and profound―but that achieves this effect by being ambiguous. On one reading it is manifestly false, but it would be earth-shaking if it were true; on the other reading it is true but trivial. The unwary listener picks up the glimmer of truth from the second reading, and the devastating importance from the first reading…. (P. 56)
Unfortunately, some statements made by philosophers are deepities.
Sources:
- Daniel C. Dennett, Intuition Pumps and Other Tools for Thinking (2013)
- Robert J. Fogelin & Walter Sinnott-Armstrong, Understanding Arguments (Fourth edition, 1991), pp. 41-42, 57
September 3rd, 2013 (Permalink)
Siegfried’s Significant Statistics Problem
Continuing the theme of what's wrong with research, Tom Siegfried, a former editor-in-chief of Science News, has an intriguing but disappointing article in the latest issue of Nautilus magazine. It starts out and ends well enough, but where's the beef? There's no meat in the middle. He does a decent job of setting up the problem, but then goes wrong in suggesting that it "has its roots in the math used to analyze the probability of experimental data" and that "even research conducted strictly by the book frequently fails because of faulty statistical methods that have been embedded in the scientific process". This is a strong and striking claim, but the arguments in the middle of the article that are apparently meant to support it are weak and confused.
After some potted history of probability theory and statistics, Siegfried tries to explain what went wrong:
At the heart of the problem is the simple mathematical hitch that a P value really doesn’t mean much. It’s just a measure of how unlikely your result is if there is no real effect. “It doesn’t tell you anything about whether the null hypothesis is true,” Gigerenzer points out. …It’s like flipping coins. Sometimes you’ll flip a penny and get several heads in a row, but that doesn’t mean the penny is rigged. Suppose, for instance, that you toss a penny 10 times. A perfectly fair coin (heads or tails equally likely) will often produce more or fewer than five heads. In fact, you’ll get exactly five heads only about a fourth of the time. Sometimes you’ll get six heads, or four. Or seven, or eight. In fact, even with a fair coin, you might get 10 heads out of 10 flips (but only about once for every thousand 10-flip trials).
So how many heads should make you suspicious? Suppose you get eight heads out of 10 tosses. For a fair coin, the chances of eight or more heads are only about 5.5 percent. That’s a P value of 0.055, close to the standard statistical significance threshold. Perhaps suspicion is warranted. But the truth is, all you know is that it’s unusual to get eight heads out of 10 flips. The penny might be weighted to favor heads, or it might just be one of those 55 times out of a thousand that eight or more heads show up. There’s no logic in concluding anything at all about the penny.
Before I start hacking my way through this thicket of confusions, let me point out that I'm not a statistician or expert in probability theory. However, as far as I can tell, this is a philosophical or logical argument rather than a technical one in mathematics. Specifically, it seems to be a version of what in philosophy is called "the problem of induction".
Exactly what the problem with induction is supposed to be is hard to pin down, but it generally involves a lack of confidence in inductive reasoning. It's the nature―even the definition―of induction that the premisses may be true and yet the conclusion false. This means that in any inductive argument, even the strongest, there's always the danger of going wrong. For this reason, philosophers have worried about induction in a way that they haven't about deduction, which is why there's no "problem of deduction". When, at the end of this passage, Siegfried says that "there’s no logic in concluding anything at all about the penny", this would seem to be what he's getting at, though it's far from clear. The fact that there is a small chance of a fair coin producing ten heads in a row is apparently enough reason, according to Siegfried, to avoid drawing any conclusion at all about the coin.
In other words, the "problem" with induction is that it's not deductive. However, the supposed philosophical "problem of induction" is insoluble, since induction can't be deductive any more than a square can be round. Siegfried tries to anticipate this response in the next paragraph:
Ah, some scientists would say, maybe you can’t conclude anything with certainty. But with only a 5 percent chance of observing the data if there’s no effect, there’s a 95 percent chance of an effect―you can be 95 percent confident that your result is real. The problem is, that reasoning is 100 percent incorrect. For one thing, the 5 percent chance of a fluke is calculated by assuming there is no effect. If there actually is an effect, the calculation is no longer valid. Besides that, such a conclusion exemplifies a logical fallacy called “transposing the conditional.” As one statistician put it, “it’s the difference between I own the house or the house owns me.”
When Siegfried writes that "the 5 percent chance of a fluke is calculated by assuming there is no effect", I guess that he's referring to the use of a null hypothesis in hypothesis testing. However, the percentage referred to is the probability of an experimental result occurring by pure chance, and whether there is an effect or not is irrelevant to that calculation. Suppose the penny in question were a two-headed coin; that has nothing to do with the probability of a fair coin producing ten heads in a row, which remains the same, since the penny is not a fair coin.
There really is a logical fallacy of “transposing the conditional”, and I have an entry for it, though not under that name (see the first Fallacy, below). However, the example given by the anonymous statistician is not a case of transposing a conditional, since there's no conditional involved. If there's a fallacy in that example it's the mistake of treating an asymmetric relation as if it were symmetric, since ownership is an asymmetric relation, that is, if A owns B then B does not own A.
Moving on:
For a simple example, suppose that each winter, I go swimming only three days―less than 5 percent of the time. In other words, there is less than a 5 percent chance of my swimming on any given day in the winter, corresponding to a P value of less than 0.05. So if you observe me swimming, is it therefore a good bet (with 95 percent confidence) that it’s not winter? No! Perhaps the only time I ever go swimming is while on vacation in Hawaii for three days every January. Then there’s less than a 5 percent chance of observing me swim in the winter, but a 100 percent chance that it is winter if you see me swimming.That’s a contrived example, of course, but it does expose a real flaw in the standard statistical methods. Studies have repeatedly shown that scientific conclusions based on calculating P values are indeed frequently false.
Other than the insistence that any chance of being wrong at all is sufficient to reject the argument―that is, the demand that the argument be deductive rather than inductive―what does this example show? One thing it shows is the importance in induction of "the requirement of total evidence": when we reason inductively, we must include among our premisses all relevant evidence, which is an additional way in which induction differs from deduction. The fact that Siegfried swims only in winter is relevant evidence, and if we fail to include it we may go wrong.
But, you may ask, how can we be sure that we've included all the relevant evidence? What if we don't even know what all the evidence is?
We can't be sure, so get used to it! Inductive reasoning is by its nature a risky endeavor: it's for the thrill-seeker, not the faint of heart. Modern philosophers, ever since Descartes, have been cautious types rather than gamblers. But deduction alone can give us knowledge only of logic and mathematics; if we want to gain any knowledge of the empirical world we live in, we have to be brave enough to take the inductive leap. We have to take some risk of being wrong, since that risk is the price that we must pay to learn about the world around us.
So, other than the fact that induction is not deductive, what's wrong with the statistics used in modern scientific research? In the remaining part of the article, Siegfried discusses such phenomena as the multiple comparisons fallacy, the "file drawer effect", publication bias, and the importance of replication―all of which we've discussed here previously (see the first Resource, below, for the most recent example)―all of which are important, but are not flaws in statistics. Instead, they are social problems of how scientific research is conducted.
Despite the deficiencies of Siegfried's arguments, it's good to see additional attention given to the problems of research. Perhaps the level of attention will soon reach a critical mass that will lead to changes in publication practice favoring replication, stricter and better-rewarded peer review, and less pressure to publish poor research. More likely, the current situation will be allowed to worsen until a serious scandal significantly lessens public confidence in, and support for, science.
Source: Tom Siegfried, "Science’s Significant Stats Problem", Nautilus, Issue 004. See also the comments at the end of the article, which are of unusually high quality and interest.
Resources:
- Check it Out, 8/29/2013
- (Added: 9/4/2013): Steven Novella, "Statistics in Science", Neurologica Blog, 3/19/2013. I found this article after having written the above. Novella seems to interpret Siegfried as arguing in favor of a Bayesian approach to research statistics, which I'm completely in sympathy with. However, Siegfried doesn't so much as mention Bayes or his famous theorem in a lengthy article on probability and statistics. If Novella is right, in attacking "the problem of induction" above I'm attacking a straw man. Nonetheless, I'll leave that attack in place because I'm not sure that Novella is correct and I think the attack on the "problem" has interest in its own right. In any case, Novella's interpretation of Siegfried is worth checking out for its own sake.
Fallacies:
Update (9/6/2013): The article discussed above seems to be a shortened version of a longer Science News article by the same author (see the Source, below), and it looks as though some of the arguments were so shortened that they no longer made sense. However, even in the longer article Siegfried at times writes as though he thinks there's something wrong with the math, whereas most if not all of his evidence comes from ways in which statistics is misinterpreted or misused. Moreover, in places he sounds as though any possibility of being wrong is sufficient reason to refrain from drawing a conclusion, for instance:
…[I]n fact, there’s no logical basis for using a P value from a single study to draw any conclusion. If the chance of a fluke is less than 5 percent, two possible conclusions remain: There is a real effect, or the result is an improbable fluke. Fisher’s method offers no way to know which is which.
In other words, there's a 5% chance of a fluke―that appears to be enough to render it "improbable"―which means there's a 95% chance of a "real effect": Siegried tells us that these are the only "two possible conclusions", and the probabilities of exclusive alternatives must add up to 100%. Yet, according to Siegfried, "there’s no logical basis…to draw any conclusion". I don't know about Fisher's method, but I know how I'm going to bet!
What does Siegfried mean by a "logical basis"? I'm not sure, but I think that he must mean a basis in deductive logic, because there's nothing in deduction that tells you which of two exclusive alternatives to choose, assuming that you can't prove or rule one out. But to insist that we can't draw any conclusion from these lopsided probabilities is just deductive chauvinism. Not only that, but later in the same article he writes:
Some studies show dramatic effects that don’t require sophisticated statistics to interpret. If the P value is 0.0001―a hundredth of a percent chance of a fluke―that is strong evidence….
This reminds me of the old joke in which a man asks a woman if she would sleep with him for a million dollars and she says "Of course!" Then, he asks if she will sleep with him for twenty bucks, and she says "No! What do you think I am?" And the man replies: "We've established that. Now we're haggling over the price."
So, now we're haggling over the level of significance: Siegfried thinks that .05 is too high but that .0001 is dandy. However, while .0001 is certainly stronger evidence than .05, that doesn't mean that .05 is no evidence at all; rather, .05 is weak evidence, but it's still evidence. Nonetheless, it might be a good idea to lower the threshold of significance to somewhere between .05 and .0001 in order to counteract the file drawer effect, publication bias, multiple comparisons, and so on.
One thing the longer article does at least partially clear up is the confusing paragraph that I quoted above where Siegfried mentions "transposing the conditional". Here's the corresponding part of the longer article:
Correctly phrased, experimental data yielding a P value of .05 means that there is only a 5 percent chance of obtaining the observed (or more extreme) result if no real effect exists…. But many explanations mangle the subtleties in that definition. A recent popular book on issues involving science, for example, states a commonly held misperception about the meaning of statistical significance at the .05 level: “This means that it is 95 percent certain that the observed difference between groups, or sets of samples, is real and could not have arisen by chance.”That interpretation commits an egregious logical error (technical term: “transposed conditional”): confusing the odds of getting a result (if a hypothesis is true) with the odds favoring the hypothesis if you observe that result. A well-fed dog may seldom bark, but observing the rare bark does not imply that the dog is hungry. A dog may bark 5 percent of the time even if it is well-fed all of the time.
Also, there's a link at the end of this section to "Box 2", which is a sidebar that explains this point in more detail. It's clear from this longer discussion that Siegfried is dealing with conditional probabilities, that is, the P value is the conditional probability of getting a certain result, r, if the null hypothesis, h, is true, or "P(r|h)" in symbols, which is not the same as the conditional probability that h is true given r, or "P(h|r)". That is, P(r|h) ≠ P(h|r), which is certainly true as a general matter.
The name "fallacy of the transposed conditional" appears to be a recent one coined by Stephen Ziliak and Deirdre McCloskey (Z&M) in their 2008 book The Cult of Statistical Significance. It also appears to be the same mistake that is sometimes called "the prosecutor's fallacy" in legal contexts, though Z&M make no mention of this in their book―at least, it's not listed in the index; I haven't read the entire book yet. This mistake is a probabilistic version of the formal fallacy of commuting a conditional, which I mentioned above, because it involves confusing the antecedent and consequent of a conditional probability. All of the formal fallacies involving conditional statements―such as affirming the consequent, denying the antecedent, and so on―involve getting the antecedent and consequent mixed up, so it makes good sense that there would be a probabilistic version of this type of fallacy, and "transposed conditional" is a better name than "the prosecutor's fallacy".
It also appears that Siegfried gets the notion that there's something wrong with the math of statistics, and not just with sloppy research practices, from Z&M's book. Since I haven't read the whole book yet, I can't comment on the nature of their claims or the cogency of their arguments. However, I think Siegfried muddies the waters by mentioning a lot of problems with research―such as low-powered studies, the file drawer effect, multiple comparisons, etc.―that have nothing to do with the statistics that Z&M criticize. I'll probably have more to say about the book after I've read it.
Sources:
- Tom Siegfried, "Odds Are, It's Wrong", Science News, 3/27/2010
- Stephen T. Ziliak & Deirdre N. McCloskey, The Cult of Statistical Significance: How the Standard Error Costs Us Jobs, Justice, and Lives (2008), pp. 17, 39, 41, 59, 73, 150-153, 155-163, 245
Answer to a Charts & Graphs Pop Quiz: This chart has two main problems:
- It's a "gee-whiz bar graph": The "bars" in the chart are, of course, the little men, but the scale to the left begins at about 158-159 centimeters, so that the chart is truncated. As a result, the little men seem to about double in height over the course of a century, whereas the scale shows an increase in height of only around ten centimeters, which is about a 6% increase.
- It's also a "one-dimensional pictograph": As the little men grow taller they grow wider, so that while the last little man is about twice as tall as the first one, his area is more like four times the area of the first. As a result, at a casual glance the viewer of the graph may get the impression that height has more than doubled over a century.
Source: Kaiser Fung, "The incredibly expanding male", Junk Charts, 9/11/2013
Solution to the Puzzle: Alice was the only sister telling the truth.
A systematic way to solve this puzzle is to look at each possible way in which the three statements could be true or false, eliminating all those that are impossible. There are only eight such possibilities, so it's doable.
However, you can eliminate the possibility that all three sisters told the truth, since each one accuses at least one of the others of not telling the truth. Is it possible that two of the sisters told the truth? If Edith told the truth then the other two sisters did not; so if two sisters told the truth it would have to be Lorie and Alice who did so. However, Lorie said that Alice would not tell the truth, so they can't both have told the truth. Therefore, only one of the sisters told the truth. Could Lorie be the only one who told the truth? No, because if Edith did not tell the truth then what Alice said was true. Could Edith be the only one telling the truth? No, because if Alice did not tell the truth then what Lorie said was true. This leaves Alice as the sole truth-teller.
This puzzle is based on one from Lewis Carroll's diary that was apparently never published during his lifetime. I have dressed it up a little from the sketchy form that it took in the diary entry. The names of the three sisters are based on the three Liddell sisters: Lorina ("Lorie"), Alice, and Edith. Alice Liddell, of course, was the basis for the character of Alice from Alice in Wonderland, the story of which was originally told by Carroll to the three sisters during an afternoon boat ride.
Sources:
- Lewis Carroll, with an introduction and notes by Martin Gardner, The Annotated Alice (1960), p. 21
- Martin Gardner, New Mathematical Diversions from Scientific American (1983), pp. 50 & 54